AI Applications in Business
By Meghan Anzelc, Ph.D., Principal, Three Arc Advisory and Christina Fernandes-D’Souza, Director of Data Science, Three Arc Advisory
What is Artificial Intelligence?
Artificial Intelligence (AI) is a concept that has been around for decades. In 1950, Alan Turing speculated about “thinking machines.” John McCarthy[1] first coined the term artificial intelligence in 1956 when he invited a group of researchers from a variety of disciplines to a summer workshop called the Dartmouth Summer Research Project on Artificial Intelligence to discuss what would ultimately become the field of AI. Nowadays, The Encyclopedia Britannica[2] states, “artificial intelligence (AI) is the ability of a digital computer or computer-controlled robot to perform tasks commonly associated with intelligent beings.” Intelligent beings are those that can adapt to changing circumstances.
In November of 2022, OpenAI launched ChatGPT, a public-facing generative AI text application. In March of 2023, Google launched its experimental version called Bard. Below is an overview of AI broadly, and how Machine Learning and Generative AI differ, generated by the Generative AI tool beautiful.ai. In the remainder of this article, we’ll share examples of how Generative AI can be used in two sectors, Insurance, and Executive Search.
Prompt Engineering
AI Generated Slide: The Basics of AI, ML, and Generative AI
How can we leverage the capabilities of generative AI like Open AI’s ChatGPT or Google’s Bard? Prompt engineering[1] is both an AI engineering technique for refining large language models (LLMs) with specific prompts and recommended outputs and the term is also used for the process of refining input to various generative AI services to generate text or images.
Principles of Prompting[2]
- Write clean and specific instructions
- Give the model time to think
- Specify the steps to complete a task (e.g., Summarize into two sentences, generate a list of three….)
- Instruct the model to work out its own solution before rushing to a conclusion
Model limitations[3]
It doesn’t always know the boundaries of its knowledge and may try to answer questions on obscure topics. It can make fabricated statements that are not true but plausible, called hallucinations. We can reduce hallucinations by asking the model to first find relevant information, then answer the question.
Some common themes we see in applications of AI in Business across different industries are automating routine tasks, enhancing and creating a customized user experience, and predicting everything from how likely you are to pay your credit card bill to what sales advertisement to display to you. We will highlight some specific use cases of Generative AI in financial services and professional services.
Generative AI in Insurance
In the spirit of this article, we asked Google’s Bard (experimental version) “Write the opening paragraph for an article about how transformative generative AI will be for insurance."
Output from Google's Bard
For insurance, we thought a really important use case to apply Generative AI would be in the claims management process. A high-level illustration of the Claims Process is provided below, generated by beautiful.ai.
AI Generated Slide: Understanding the Car Insurance Claims Process
A claimant needs to collect and provide numerous documents when they file a claim - accident report, medical bills, pictures/videos of damage, etc. The claims adjuster will read all the documentation and enter all relevant information into the system in the form of claim notes. Next, the claims adjuster may do their own investigation, determine coverage, arrange for repair or replacement of the vehicle and provide payment.
AI Generated Slide: How AI and ML Can Transform the Auto Claims Process
The following is where we see Generative AI being applied to the claim’s workflow process:
- Expediting the claims process by summarizing and inferring crucial information from countless numbers of documents into claims notes for the claims adjuster to review. With prior approaches, it was typically impractical to sift through all information available and surface relevant data.
- Claims Processing Automation by “triaging” the claims based on parameters that will allow the system to better automatically close out low-value claims, send high-severity claims to an experienced claims adjuster, or redirect the claim to fraud investigators. While this has been done using more traditional AI/ML approaches in the past, there is likely an opportunity to use Generative AI to make better use of all the information available to refine current approaches.
- Fraud detection - it has been estimated that the Insurance Industry loses approximately $308 billion annually due to fraudulent claims. While AI is currently being used for fraud detection in claims data, the amount of viable fraud sample data points is quite low and as a result difficult for machine learning algorithms to learn from. Generative AI can generate new, synthetic samples that are like ‘real’ fraud samples, boosting the fraud signal for core fraud detection machine learning tools.
- Providing exceptional Customer Service - chatbots can be used effectively to customize a customer’s experience while going through the claims process. This quick and efficient support will improve customer satisfaction. Better identifying when a customer should be routed to an experienced claims adjuster, such as when they have just been through a devastating loss, can be addressed by a mix of Generative AI to converse with a customer and better AI/ML approaches to identify when human intervention is needed.
Generative AI in Executive Search
Similarly, we prompted ChatGPT-4 (on forefront.ai) “Write the opening paragraph for an article about how transformative generative AI will be for Executive Search”
There is an enormous amount of opportunity to reduce bias in Executive Search. Some of the biases we have seen are gender bias, pedigree bias, similarity bias, location bias, recency bias, and affinity bias, to name a few.
Identifying and sourcing top talent in Executive Search is vital for business and is challenging in Executive Search since the data is nuanced and thin. Candidate matching and ranking involves using different types of data like structured, semi-structured, and unstructured data from job descriptions, resumes, candidate reports, internal comments from interviews and candidate feedback, company profiles, etc.
Where Generative AI can make a significant difference is in digesting the data available to make patterns more readily available. Previously, for every task like extracting relevant information and performing sentiment analysis, you had to collect labeled data, train and deploy a separate model to make inferences. With Generative AI, you only need to write effective prompts and results can be generated right away, reducing a lot of time and effort involved in developing different workstreams for different tasks, thus allowing you to quickly develop an effective candidate matching system paying attention to unconscious biases.
This means that even smaller boutique Executive Search firms have the opportunity to take advantage of these capabilities at scale and at a reasonable cost, offering the possibility of significant competitive advantage to those who move first to embrace and embed AI into their business processes.
Summary
These are just a few example applications of how Generative AI can be used in just two industries. There are significant opportunities for these capabilities to add value across a wide range of use cases. As with every analytics endeavor, ask yourself some guiding questions before you embark on the Generative AI journey:
- What are you trying to solve?
- Is Generative AI an appropriate solution?
- Have you evaluated different tools, and the different outcomes from using these tools?
- Have you read and assessed the different user agreements around these tools? Pay extra attention to providing proprietary data to these tools.
- What are the risks of using Generative AI? Can they be mitigated? Some examples are incorporating unintended biases, copyright infringement and lack of attribution of source material, inaccurate outputs also called "hallucinations", unintended disclosure of proprietary or confidential information, and unintended non-compliance with regulation.
- Monitoring and measuring the outcomes of these tools, and asking yourself does it add value.
We have been impressed by very early results from Generative AI tools and how they can be applied to business problems in practical ways. As these capabilities continue to evolve, it will be important for organizations to start considering when to build their own Generative AI tools in-house and when to partner and use a vendor solution such as Open AI’s ChatGPT or Google’s Bard. Those who find ways to take advantage of early opportunities to embrace this new and exciting area of AI will build a competitive advantage.
[1] https://www.livinginternet.com/i/ii_ai.htm
[2] https://www.britannica.com/technology/artificial-intelligence
[3] https://bit.ly/44nbdQe
[4] https://www.deeplearning.ai/short-courses/chatgpt-prompt-engineering-for-developers/
[5] https://www.deeplearning.ai/short-courses/chatgpt-prompt-engineering-for-developers/
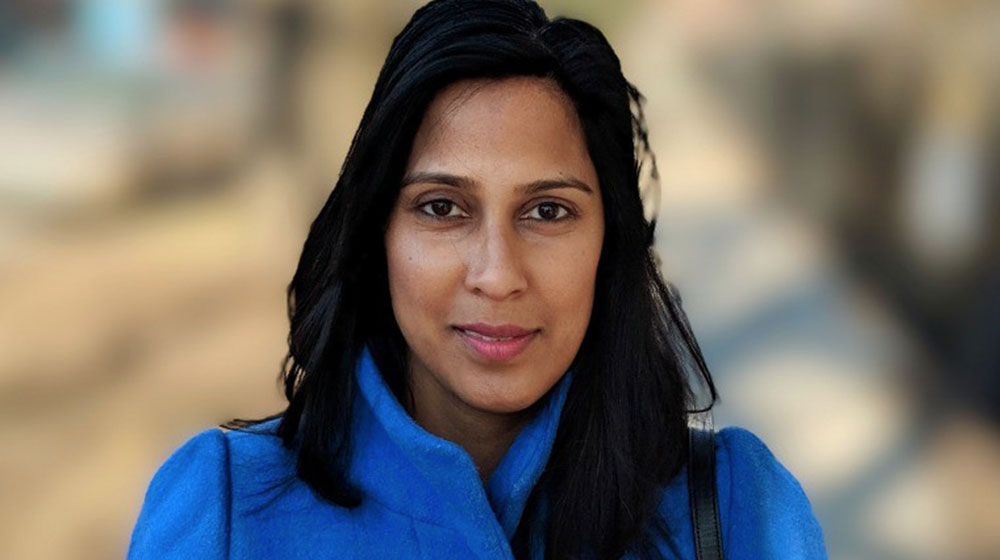
About the author
Christina Fernandes-D’Souza has over 15 years of global, cross-industry experience, in data and analytics, combining strategy, data, analytics, machine learning and technology, in both the private and public sectors. She holds a Master’s in Applied Mathematics.
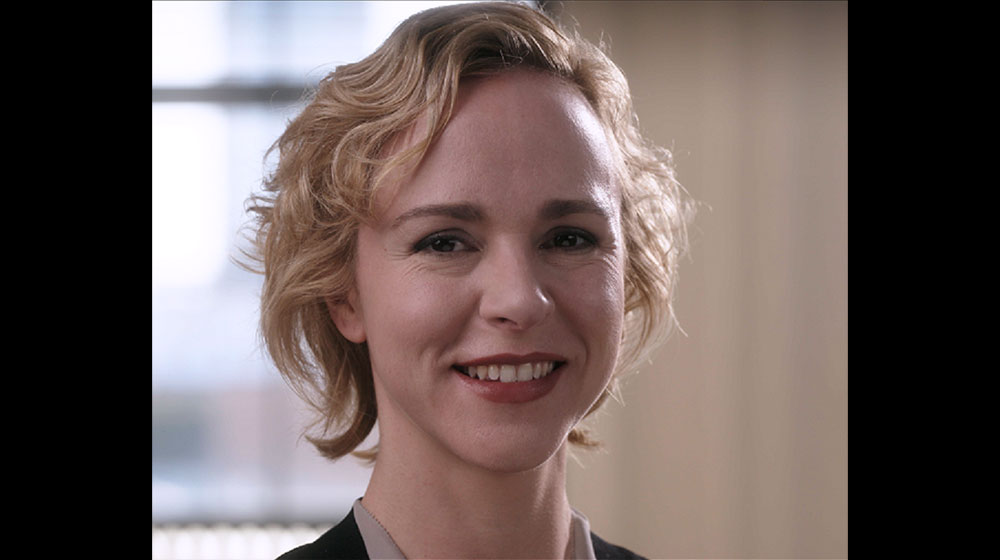
About the author
Dr. Meghan Anzelc has nearly two decades of experience driving impact through the use of data, AI, and technology. Previously Head of Data & Analytics at Spencer Stuart and Chief Analytics Officer at AXIS Capital, she was brought into both organizations to build analytics functions from 0-1. Dr. Anzelc previously held multiple leadership roles in the insurance industry and was a board member of the Chicago Literacy Alliance. She holds a MS and PhD in Physics from Northwestern University and a BS in Physics from Loyola University Chicago.
By Meghan Anzelc, Ph.D., Principal, Three Arc Advisory and Christina Fernandes-D’Souza, Director of Data Science, Three Arc Advisory
What is Artificial Intelligence?
Artificial Intelligence (AI) is a concept that has been around for decades. In 1950, Alan Turing speculated about “thinking machines.” John McCarthy[1] first coined the term artificial intelligence in 1956 when he invited a group of researchers from a variety of disciplines to a summer workshop called the Dartmouth Summer Research Project on Artificial Intelligence to discuss what would ultimately become the field of AI. Nowadays, The Encyclopedia Britannica[2] states, “artificial intelligence (AI) is the ability of a digital computer or computer-controlled robot to perform tasks commonly associated with intelligent beings.” Intelligent beings are those that can adapt to changing circumstances.
In November of 2022, OpenAI launched ChatGPT, a public-facing generative AI text application. In March of 2023, Google launched its experimental version called Bard. Below is an overview of AI broadly, and how Machine Learning and Generative AI differ, generated by the Generative AI tool beautiful.ai. In the remainder of this article, we’ll share examples of how Generative AI can be used in two sectors, Insurance, and Executive Search.
Prompt Engineering
How can we leverage the capabilities of generative AI like Open AI’s ChatGPT or Google’s Bard? Prompt engineering[1] is both an AI engineering technique for refining large language models (LLMs) with specific prompts and recommended outputs and the term is also used for the process of refining input to various generative AI services to generate text or images.
Principles of Prompting[2]
- Write clean and specific instructions
- Give the model time to think
- Specify the steps to complete a task (e.g., Summarize into two sentences, generate a list of three….)
- Instruct the model to work out its own solution before rushing to a conclusion
Model limitations[3]
It doesn’t always know the boundaries of its knowledge and may try to answer questions on obscure topics. It can make fabricated statements that are not true but plausible, called hallucinations. We can reduce hallucinations by asking the model to first find relevant information, then answer the question.
Some common themes we see in applications of AI in Business across different industries are automating routine tasks, enhancing and creating a customized user experience, and predicting everything from how likely you are to pay your credit card bill to what sales advertisement to display to you. We will highlight some specific use cases of Generative AI in financial services and professional services.
Generative AI in Insurance
In the spirit of this article, we asked Google’s Bard (experimental version) “Write the opening paragraph for an article about how transformative generative AI will be for insurance."
For insurance, we thought a really important use case to apply Generative AI would be in the claims management process. A high-level illustration of the Claims Process is provided below, generated by beautiful.ai.
A claimant needs to collect and provide numerous documents when they file a claim - accident report, medical bills, pictures/videos of damage, etc. The claims adjuster will read all the documentation and enter all relevant information into the system in the form of claim notes. Next, the claims adjuster may do their own investigation, determine coverage, arrange for repair or replacement of the vehicle and provide payment.
The following is where we see Generative AI being applied to the claim’s workflow process:
- Expediting the claims process by summarizing and inferring crucial information from countless numbers of documents into claims notes for the claims adjuster to review. With prior approaches, it was typically impractical to sift through all information available and surface relevant data.
- Claims Processing Automation by “triaging” the claims based on parameters that will allow the system to better automatically close out low-value claims, send high-severity claims to an experienced claims adjuster, or redirect the claim to fraud investigators. While this has been done using more traditional AI/ML approaches in the past, there is likely an opportunity to use Generative AI to make better use of all the information available to refine current approaches.
- Fraud detection - it has been estimated that the Insurance Industry loses approximately $308 billion annually due to fraudulent claims. While AI is currently being used for fraud detection in claims data, the amount of viable fraud sample data points is quite low and as a result difficult for machine learning algorithms to learn from. Generative AI can generate new, synthetic samples that are like ‘real’ fraud samples, boosting the fraud signal for core fraud detection machine learning tools.
- Providing exceptional Customer Service - chatbots can be used effectively to customize a customer’s experience while going through the claims process. This quick and efficient support will improve customer satisfaction. Better identifying when a customer should be routed to an experienced claims adjuster, such as when they have just been through a devastating loss, can be addressed by a mix of Generative AI to converse with a customer and better AI/ML approaches to identify when human intervention is needed.
Generative AI in Executive Search
Similarly, we prompted ChatGPT-4 (on forefront.ai) “Write the opening paragraph for an article about how transformative generative AI will be for Executive Search”
There is an enormous amount of opportunity to reduce bias in Executive Search. Some of the biases we have seen are gender bias, pedigree bias, similarity bias, location bias, recency bias, and affinity bias, to name a few.
Identifying and sourcing top talent in Executive Search is vital for business and is challenging in Executive Search since the data is nuanced and thin. Candidate matching and ranking involves using different types of data like structured, semi-structured, and unstructured data from job descriptions, resumes, candidate reports, internal comments from interviews and candidate feedback, company profiles, etc.
Where Generative AI can make a significant difference is in digesting the data available to make patterns more readily available. Previously, for every task like extracting relevant information and performing sentiment analysis, you had to collect labeled data, train and deploy a separate model to make inferences. With Generative AI, you only need to write effective prompts and results can be generated right away, reducing a lot of time and effort involved in developing different workstreams for different tasks, thus allowing you to quickly develop an effective candidate matching system paying attention to unconscious biases.
This means that even smaller boutique Executive Search firms have the opportunity to take advantage of these capabilities at scale and at a reasonable cost, offering the possibility of significant competitive advantage to those who move first to embrace and embed AI into their business processes.
Summary
These are just a few example applications of how Generative AI can be used in just two industries. There are significant opportunities for these capabilities to add value across a wide range of use cases. As with every analytics endeavor, ask yourself some guiding questions before you embark on the Generative AI journey:
- What are you trying to solve?
- Is Generative AI an appropriate solution?
- Have you evaluated different tools, and the different outcomes from using these tools?
- Have you read and assessed the different user agreements around these tools? Pay extra attention to providing proprietary data to these tools.
- What are the risks of using Generative AI? Can they be mitigated? Some examples are incorporating unintended biases, copyright infringement and lack of attribution of source material, inaccurate outputs also called "hallucinations", unintended disclosure of proprietary or confidential information, and unintended non-compliance with regulation.
- Monitoring and measuring the outcomes of these tools, and asking yourself does it add value.
We have been impressed by very early results from Generative AI tools and how they can be applied to business problems in practical ways. As these capabilities continue to evolve, it will be important for organizations to start considering when to build their own Generative AI tools in-house and when to partner and use a vendor solution such as Open AI’s ChatGPT or Google’s Bard. Those who find ways to take advantage of early opportunities to embrace this new and exciting area of AI will build a competitive advantage.
[1] https://www.livinginternet.com/i/ii_ai.htm
[2] https://www.britannica.com/technology/artificial-intelligence
[3] https://bit.ly/44nbdQe
[4] https://www.deeplearning.ai/short-courses/chatgpt-prompt-engineering-for-developers/
[5] https://www.deeplearning.ai/short-courses/chatgpt-prompt-engineering-for-developers/