How To Transform Retail Supply and Demand Planning With AI
By Sam Iosevich
Chief Analytic Officer and Manager Partner, Parker Avery Group
Artificial intelligence (AI) has the opportunity to dramatically transform retail and consumer brand organizations. Yet many are struggling to understand the best deployment scenarios that will provide a justifiable return on investment. Further, traditional retail organizations and the systems that support their operations are often hampered by mindsets and platforms that hamper effective collaboration. In this article, we unpack some of these challenges and outline the vision and enablers for how AI can be the transformational silver bullet for retail planning.
Drawbacks of most modern planning systems
From merchandise planning and pricing to fulfillment and operations, retailers have a plethora of tools available to assist in supporting their operations, and, by most measures, they work. While these tools can be powerful, there is a severe drawback. Because they all have built-in analytics that provide a singular interpretation of demand, they impede collaboration outside of their functional area. The issue is this interpretation is neither consistent nor typically shared across other functional areas. By default, these tools create siloed operations across functional areas, hindering any ability to have a consistent understanding of demand needs. Further, these silos with their multiple demand signals create roadblocks in seamlessly linking demand to a balanced supply response. The result is a lack of fluidity and agility in operational orchestration across the broader organization.
Connected and collaborative supply chain functions
The vision and goal are to go from siloed functions to a connected ecosystem. Foundational to this connected ecosystem is the ability to tie demand needs with a balanced supply response. Doing so establishes a shared and consistent understanding of unconstrained demand and organizational risks and constraints, actioned by seamless collaboration within and across functional areas.
Enterprise Demand Graph
By centralizing and sharing clean and consistent data with AI-powered predictive insights, the organization becomes empowered with a highly accurate and common interpretation of the demand potential. Further, visibility into demand drivers enables congruent and optimized actions within the confines of constraints. Embedding analytics into this process can transform the organization from managing the business via a singular functional view to an expansive enterprise view that, if done correctly, drives operational efficiency. AI is the cornerstone of this enablement.
So where exactly does AI play a part in creating an agile and connected ecosystem that enables organizations to have a common view of demand needs and align that with a balanced supply response? It starts with a common AI-driven demand forecasting foundation.
The holistic AI-driven supply and demand planning capability
By establishing an AI-driven demand forecasting foundation, organizations can achieve real-time concurrent harmonization and improvement across all business functions within the enterprise. Incorporating key considerations and other AI, ML, and reinforcement learning techniques, a well-constructed AI demand forecasting foundation not only infuses existing systems and business processes with greater intelligence but also establishes common “facts” that empower constituents to collaborate.
The result is systems and operational processes that are significantly more intelligent and informed than they otherwise would be. For more progressive capabilities, “what-if” capabilities can be created, allowing end users to test various inputs and outcomes via a simulation engine. With each simulation iteration, stakeholders can view and collaborate in real-time on different scenarios that balance and optimize opportunity with organizational risks and constraints.
AI Demand Forecasting Graph
Key AI capabilities that drive the connected retail ecosystem
As retailers venture to transform their operations, the following are some high-level considerations in the deployment of an AI-infused demand forecasting foundation.
- Automated data cleansing with anomaly detection detects irregularities in the data and either removes that data component, fixes it, or generates an alert for the user to intervene.
The key to data cleansing is to have a broad set of filters that sift through sales and demand drivers to ensure that erroneous data does not lead to errors in predictions. Machine learning should be used to continuously monitor statistical distributions of the data, detecting level shifts and other irregularities, such as alterations in seasonality and trend as they start to develop. Also, as data is being federated, the use of AI should be considered to auto-detect inconsistencies in the data, such as attribution, to ensure all related data is common and consistent. - Decomposition of the demand signal using AI/ML to isolate base demand from other demand components and their effects.
For example, AI can and should be used to separate the various components of the total demand signal, such as base, incremental, seasonality/trend, cannibalization, and halo. By isolating these components, we can better explain various internal and external influences on the demand signal, such as the impact of COVID-19, the lift associated with promotional activity, consumer behavior changes, competitive implications including cannibalization/halo effects, etc. The effects of these influences and their magnitude are saved as elasticity measures and archived to be used for estimating the impacts of future events.
- Intelligent automation to assist with the auto-selection of a champion forecast model.
Not all forecast models are created equal. In fact, it is quite common for demand patterns to differ drastically across products and locations. Therefore, it is imperative to have a comprehensive repository of configurable forecasting models that can be considered to ensure the most accurate future demand projections. Ideally, AI can be used to measure the accuracy of each model and to auto-select a champion model that produces the most accurate forecast with the least amount of bias. Some models to consider may include Uber Orbit, FB Prophet, ARIMA/ARIMAX, Exponential Smoothing, and others. - Propagation of the forecast to all intersections of product, location, and time hierarchies leveraging ML.
A primary consideration for empowering constituents to collaborate is to establish a common depiction of demand across the enterprise. ML is a perfect tool to disaggregate and reaggregate the generated forecasts to all intersections of the cube. It is imperative that the underlying architecture also be highly scalable noting vast amounts of data will undoubtedly be considered as data is propagated.
- Forecast quality and stability reporting.
After the forecast is consumed, it is important to assess its quality and identify opportunities for accuracy improvement. A set of actionable reports should be created that measure overall forecast precision and identify model degradation as part of the continuous improvement process.
Final word
When infused appropriately into business operations and well executed, AI represents a paradigm shift in how retail and consumer brand organizations think, interact, and orchestrate. In this capacity, the efficiency and productivity gains of AI-infused retail supply and demand planning can be massive.
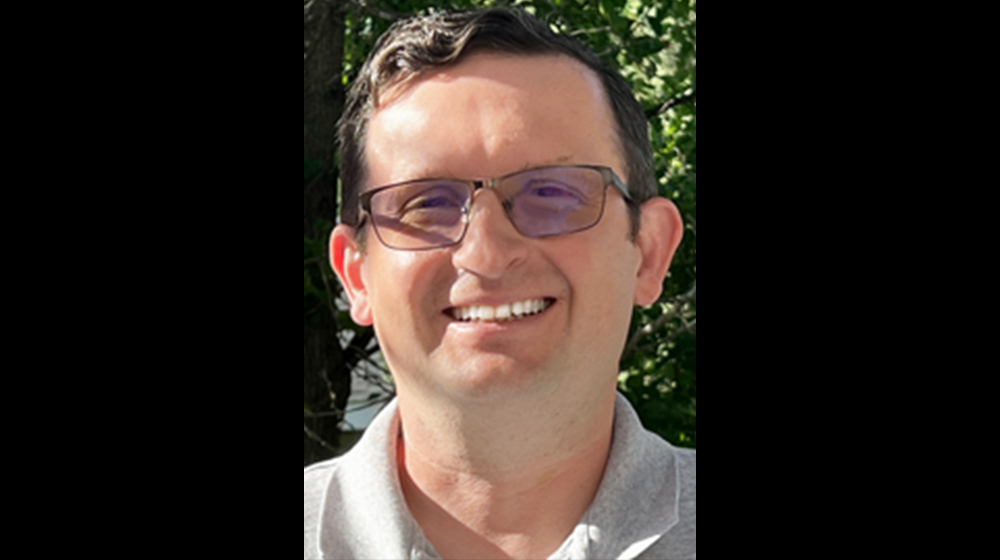
About the author
Sam Iosevich is the Chief Analytic Office and Manager Partner for the Parker Avery Group. Sam has lived in the world of Demand Forecasting and Planning for over 25 years. After selling his first company, Prognos Inc, Sam, and his team, went back to work to reimagine the art of the possible and conceived the hypercube concept – the ability to plan all corners of the organization with a common demand signal and drivers, empowering seamless collaboration within and across functional siloes, and end-to-end agile orchestration across the enterprise.
By Sam Iosevich
Chief Analytic Officer and Manager Partner, Parker Avery Group
Artificial intelligence (AI) has the opportunity to dramatically transform retail and consumer brand organizations. Yet many are struggling to understand the best deployment scenarios that will provide a justifiable return on investment. Further, traditional retail organizations and the systems that support their operations are often hampered by mindsets and platforms that hamper effective collaboration. In this article, we unpack some of these challenges and outline the vision and enablers for how AI can be the transformational silver bullet for retail planning.
Drawbacks of most modern planning systems
From merchandise planning and pricing to fulfillment and operations, retailers have a plethora of tools available to assist in supporting their operations, and, by most measures, they work. While these tools can be powerful, there is a severe drawback. Because they all have built-in analytics that provide a singular interpretation of demand, they impede collaboration outside of their functional area. The issue is this interpretation is neither consistent nor typically shared across other functional areas. By default, these tools create siloed operations across functional areas, hindering any ability to have a consistent understanding of demand needs. Further, these silos with their multiple demand signals create roadblocks in seamlessly linking demand to a balanced supply response. The result is a lack of fluidity and agility in operational orchestration across the broader organization.
Connected and collaborative supply chain functions
The vision and goal are to go from siloed functions to a connected ecosystem. Foundational to this connected ecosystem is the ability to tie demand needs with a balanced supply response. Doing so establishes a shared and consistent understanding of unconstrained demand and organizational risks and constraints, actioned by seamless collaboration within and across functional areas.
By centralizing and sharing clean and consistent data with AI-powered predictive insights, the organization becomes empowered with a highly accurate and common interpretation of the demand potential. Further, visibility into demand drivers enables congruent and optimized actions within the confines of constraints. Embedding analytics into this process can transform the organization from managing the business via a singular functional view to an expansive enterprise view that, if done correctly, drives operational efficiency. AI is the cornerstone of this enablement.
So where exactly does AI play a part in creating an agile and connected ecosystem that enables organizations to have a common view of demand needs and align that with a balanced supply response? It starts with a common AI-driven demand forecasting foundation.
The holistic AI-driven supply and demand planning capability
By establishing an AI-driven demand forecasting foundation, organizations can achieve real-time concurrent harmonization and improvement across all business functions within the enterprise. Incorporating key considerations and other AI, ML, and reinforcement learning techniques, a well-constructed AI demand forecasting foundation not only infuses existing systems and business processes with greater intelligence but also establishes common “facts” that empower constituents to collaborate.
The result is systems and operational processes that are significantly more intelligent and informed than they otherwise would be. For more progressive capabilities, “what-if” capabilities can be created, allowing end users to test various inputs and outcomes via a simulation engine. With each simulation iteration, stakeholders can view and collaborate in real-time on different scenarios that balance and optimize opportunity with organizational risks and constraints.
Key AI capabilities that drive the connected retail ecosystem
As retailers venture to transform their operations, the following are some high-level considerations in the deployment of an AI-infused demand forecasting foundation.
- Automated data cleansing with anomaly detection detects irregularities in the data and either removes that data component, fixes it, or generates an alert for the user to intervene.
The key to data cleansing is to have a broad set of filters that sift through sales and demand drivers to ensure that erroneous data does not lead to errors in predictions. Machine learning should be used to continuously monitor statistical distributions of the data, detecting level shifts and other irregularities, such as alterations in seasonality and trend as they start to develop. Also, as data is being federated, the use of AI should be considered to auto-detect inconsistencies in the data, such as attribution, to ensure all related data is common and consistent. - Decomposition of the demand signal using AI/ML to isolate base demand from other demand components and their effects.
For example, AI can and should be used to separate the various components of the total demand signal, such as base, incremental, seasonality/trend, cannibalization, and halo. By isolating these components, we can better explain various internal and external influences on the demand signal, such as the impact of COVID-19, the lift associated with promotional activity, consumer behavior changes, competitive implications including cannibalization/halo effects, etc. The effects of these influences and their magnitude are saved as elasticity measures and archived to be used for estimating the impacts of future events.
- Intelligent automation to assist with the auto-selection of a champion forecast model.
Not all forecast models are created equal. In fact, it is quite common for demand patterns to differ drastically across products and locations. Therefore, it is imperative to have a comprehensive repository of configurable forecasting models that can be considered to ensure the most accurate future demand projections. Ideally, AI can be used to measure the accuracy of each model and to auto-select a champion model that produces the most accurate forecast with the least amount of bias. Some models to consider may include Uber Orbit, FB Prophet, ARIMA/ARIMAX, Exponential Smoothing, and others. - Propagation of the forecast to all intersections of product, location, and time hierarchies leveraging ML.
A primary consideration for empowering constituents to collaborate is to establish a common depiction of demand across the enterprise. ML is a perfect tool to disaggregate and reaggregate the generated forecasts to all intersections of the cube. It is imperative that the underlying architecture also be highly scalable noting vast amounts of data will undoubtedly be considered as data is propagated.
- Forecast quality and stability reporting.
After the forecast is consumed, it is important to assess its quality and identify opportunities for accuracy improvement. A set of actionable reports should be created that measure overall forecast precision and identify model degradation as part of the continuous improvement process.
Final word
When infused appropriately into business operations and well executed, AI represents a paradigm shift in how retail and consumer brand organizations think, interact, and orchestrate. In this capacity, the efficiency and productivity gains of AI-infused retail supply and demand planning can be massive.