Supply Chain Visibility: The Key to AI Success
By Josh Mayer
Head of Professional Services, EasyPost
The convergence of cutting-edge technology with traditional supply chain practices marks the dawn of a new era in improving decision-making and operational performance. Artificial Intelligence (AI) plays a pivotal role in this transformational journey, offering the prospect of revolutionizing supply chain management for retail and consumer goods enterprises. Yet, the key to successfully implementing AI into supply chains hinges on establishing a solid foundation of supply chain visibility.
The Pivotal Role of Supply Chain Visibility
In a recent survey of more than 300 supply chain executives, "visibility" in the supply chain was closely linked with "transparency." Transparency refers to the collaboration of people, processes, and technology essential to facilitate the physical flow of goods across the supply chain.
In its ideal state, visibility incorporates comprehensive insights from every process, transaction, and product movement from raw materials to end customer. Achieving this level of visibility is no small feat and requires strong internal and external relationships and a solid understanding of the business.
In addition to solid relationships, achieving end-to-end supply chain visibility requires access to many new types of data beyond traditional transactional systems.
Internal vs. External Data
Internal data sets, such as data produced by warehouse and transportation management systems, provide insights into the operations within the company's control. External data sets, such as competitive benchmarks, weather forecasts, social media, or supplier communication, can impact supply chain performance but are not within the company's direct influence.
For instance, consider demand forecasting. AI can more accurately predict future product demand by analyzing internal data, such as historical sales and inventory turnover rates, alongside external factors like supplier performance. For a supply chain heavily reliant on supplier communication, it is only possible to operate at a high level with timely and effective communication from its suppliers.
Structured vs. Unstructured Data
Companies must also collect structured and unstructured data sets to bolster visibility initiatives. Structured data refers to highly organized information easily searchable by widely adopted and traditional methods. Typically, this encompasses databases of inventory levels, supplier performance metrics, and carrier transit times.
On the other hand, unstructured data—such as emails, images, videos, social media posts, and maintenance logs—presents a more complex challenge. While rich in insight, this data type requires advanced skill sets and tooling to parse and interpret.
For example, modern technology allows images of the warehouse yard to be captured in near real-time to help identify and track trailers and containers as they arrive. Images are an example of unstructured data that can be combined with yard management (structured) system data for new reconciliation methods and improved operational insights.
Data Validation & Organizational Alignment
Achieving a high level of supply chain visibility results in the generation of new insights. These insights, derived from analyzing structured and unstructured and internal and external data sets, can significantly impact decision-making and strategic planning. However, the emergence of these insights does not guarantee their accuracy. Therefore, it becomes crucial to validate these new findings rigorously.
Organizations should assemble cross-functional teams comprising members from supply chain, technology, merchandising, digital, finance, and other functions to analyze the vast amounts of data collected.
These teams should engage in collaborative data exploration sessions, utilizing statistical methods and visualization tools to uncover trends, correlations, and anomalies within data sets. Such a cross-functional approach leverages different stakeholders' unique perspectives and expertise and fosters a culture of data literacy and analytical thinking across the organization.
Once the teams identify patterns and potential areas of improvement through data analysis, it is vital for the organization to align on the level of readiness for AI application, set realistic expectations for its outcomes, and establish metrics for measuring success.
AI Use Cases in Supply Chain
With the proper supply chain visibility, understanding, and internal alignment, organizations can embark on the journey to implement AI capabilities.
The most common AI applications today help supply chain teams with the following use cases:
- Event Prediction: Anticipating disruptions, demand spikes, and capacity constraints, enabling proactive action to mitigate risks.
- Supplier Relationship Management: Monitoring and analyzing supplier performance data helps manage risks and develop strategic relationships.
- Demand Forecasting: Employing AI to perform complex market data analyses enhances sales forecasts' accuracy and drives efficient production planning.
- Inventory Management: Using real-time data to optimize stock levels, reduce carrying costs, and improve inventory turns.
- Transportation Logistics: Intelligent routing and shipment consolidation can substantially reduce shipping costs and improve delivery times.
Each use case demonstrates AI's transformative potential, contingent upon the depth, breadth, and quality of the data collected.
Conclusion
In summary, improving supply chain performance with AI hinges on better visibility. It combines structured and unstructured data from within and outside the organization to analyze and improve supply chain performance. Strong internal and external relationships are key to successfully implementing AI applications, such as predicting events, managing supplier relationships, forecasting demand, handling inventory, and streamlining transportation logistics.
These use cases demonstrate how AI can bring real benefits when supported by a solid foundation of visibility. Achieving AI's maximum potential in supply chain management is a challenging journey, but the payoffs can boost your company's competitiveness and operational efficiency.
Therefore, organizations must prioritize enhancing supply chain visibility. This ensures the successful deployment of AI and solidifies the groundwork for future innovations in supply chain management. The convergence of diverse data sets, a unified strategic vision, and steadfast organizational alignment heralds a new era of supply chain efficiency and resilience.
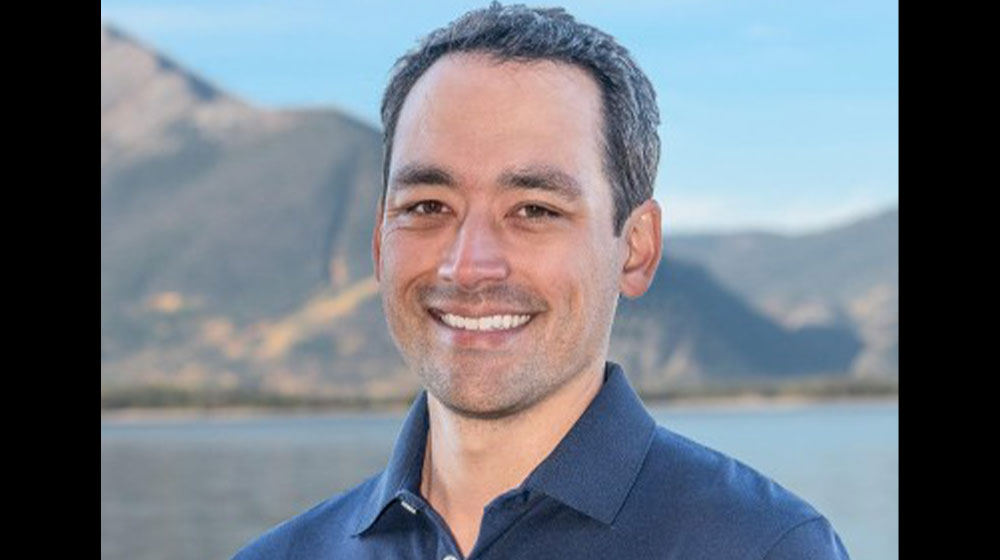
About the author
Josh Mayer is the Head of Professional Services at EasyPost, a modern supply chain solutions leader. Before joining EasyPost via acquisition in July 2022, he founded supply chain consulting and analytics firms and has helped over 100 enterprise organizations improve operational performance. Beyond work, he enjoys time with his incredible wife and two daughters, who keep him on his toes.
By Josh Mayer
Head of Professional Services, EasyPost
The convergence of cutting-edge technology with traditional supply chain practices marks the dawn of a new era in improving decision-making and operational performance. Artificial Intelligence (AI) plays a pivotal role in this transformational journey, offering the prospect of revolutionizing supply chain management for retail and consumer goods enterprises. Yet, the key to successfully implementing AI into supply chains hinges on establishing a solid foundation of supply chain visibility.
The Pivotal Role of Supply Chain Visibility
In a recent survey of more than 300 supply chain executives, "visibility" in the supply chain was closely linked with "transparency." Transparency refers to the collaboration of people, processes, and technology essential to facilitate the physical flow of goods across the supply chain.
In its ideal state, visibility incorporates comprehensive insights from every process, transaction, and product movement from raw materials to end customer. Achieving this level of visibility is no small feat and requires strong internal and external relationships and a solid understanding of the business.
In addition to solid relationships, achieving end-to-end supply chain visibility requires access to many new types of data beyond traditional transactional systems.
Internal vs. External Data
Internal data sets, such as data produced by warehouse and transportation management systems, provide insights into the operations within the company's control. External data sets, such as competitive benchmarks, weather forecasts, social media, or supplier communication, can impact supply chain performance but are not within the company's direct influence.
For instance, consider demand forecasting. AI can more accurately predict future product demand by analyzing internal data, such as historical sales and inventory turnover rates, alongside external factors like supplier performance. For a supply chain heavily reliant on supplier communication, it is only possible to operate at a high level with timely and effective communication from its suppliers.
Structured vs. Unstructured Data
Companies must also collect structured and unstructured data sets to bolster visibility initiatives. Structured data refers to highly organized information easily searchable by widely adopted and traditional methods. Typically, this encompasses databases of inventory levels, supplier performance metrics, and carrier transit times.
On the other hand, unstructured data—such as emails, images, videos, social media posts, and maintenance logs—presents a more complex challenge. While rich in insight, this data type requires advanced skill sets and tooling to parse and interpret.
For example, modern technology allows images of the warehouse yard to be captured in near real-time to help identify and track trailers and containers as they arrive. Images are an example of unstructured data that can be combined with yard management (structured) system data for new reconciliation methods and improved operational insights.
Data Validation & Organizational Alignment
Achieving a high level of supply chain visibility results in the generation of new insights. These insights, derived from analyzing structured and unstructured and internal and external data sets, can significantly impact decision-making and strategic planning. However, the emergence of these insights does not guarantee their accuracy. Therefore, it becomes crucial to validate these new findings rigorously.
Organizations should assemble cross-functional teams comprising members from supply chain, technology, merchandising, digital, finance, and other functions to analyze the vast amounts of data collected.
These teams should engage in collaborative data exploration sessions, utilizing statistical methods and visualization tools to uncover trends, correlations, and anomalies within data sets. Such a cross-functional approach leverages different stakeholders' unique perspectives and expertise and fosters a culture of data literacy and analytical thinking across the organization.
Once the teams identify patterns and potential areas of improvement through data analysis, it is vital for the organization to align on the level of readiness for AI application, set realistic expectations for its outcomes, and establish metrics for measuring success.
AI Use Cases in Supply Chain
With the proper supply chain visibility, understanding, and internal alignment, organizations can embark on the journey to implement AI capabilities.
The most common AI applications today help supply chain teams with the following use cases:
- Event Prediction: Anticipating disruptions, demand spikes, and capacity constraints, enabling proactive action to mitigate risks.
- Supplier Relationship Management: Monitoring and analyzing supplier performance data helps manage risks and develop strategic relationships.
- Demand Forecasting: Employing AI to perform complex market data analyses enhances sales forecasts' accuracy and drives efficient production planning.
- Inventory Management: Using real-time data to optimize stock levels, reduce carrying costs, and improve inventory turns.
- Transportation Logistics: Intelligent routing and shipment consolidation can substantially reduce shipping costs and improve delivery times.
Each use case demonstrates AI's transformative potential, contingent upon the depth, breadth, and quality of the data collected.
Conclusion
In summary, improving supply chain performance with AI hinges on better visibility. It combines structured and unstructured data from within and outside the organization to analyze and improve supply chain performance. Strong internal and external relationships are key to successfully implementing AI applications, such as predicting events, managing supplier relationships, forecasting demand, handling inventory, and streamlining transportation logistics.
These use cases demonstrate how AI can bring real benefits when supported by a solid foundation of visibility. Achieving AI's maximum potential in supply chain management is a challenging journey, but the payoffs can boost your company's competitiveness and operational efficiency.
Therefore, organizations must prioritize enhancing supply chain visibility. This ensures the successful deployment of AI and solidifies the groundwork for future innovations in supply chain management. The convergence of diverse data sets, a unified strategic vision, and steadfast organizational alignment heralds a new era of supply chain efficiency and resilience.